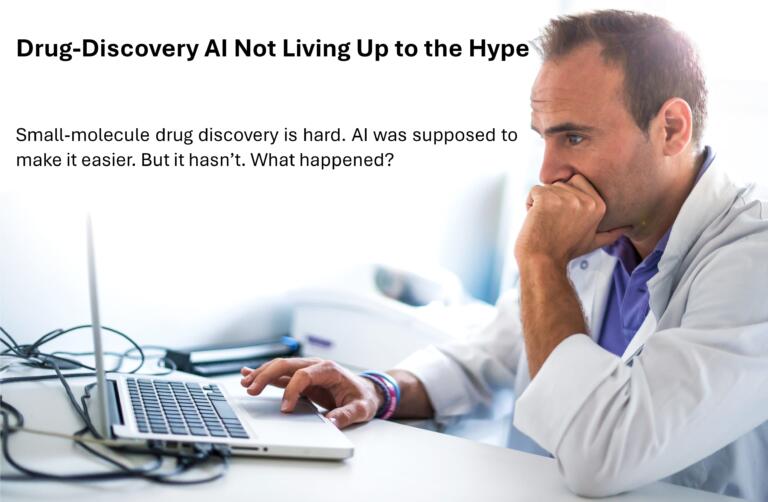
More and more people are starting to decry the lack of progress from AI-driven companies that promised large numbers of drugs would come to market by now. And what they’ve produced is neither novel nor performing well in clinical trials.
Major Failures
The largest players in the space have seen several drug candidates shelved or dropped by their partners as their drugs either fail in trials or return otherwise lackluster data. At least one of these companies hasn’t generated drug candidates using AI; they simply outlicensed drug candidates made the old-fashioned way in university labs.
Nothing New
In my recent discussion with Verseon’s CEO Adityo Prakash, I learned that the seeds of failure for the cur-rent crop of AI drug-discovery companies can be seen in their patent filings. Examining their so-called “new” drugs’ structures when compared to older drugs makes one wonder about what passes for AI driven innovation:
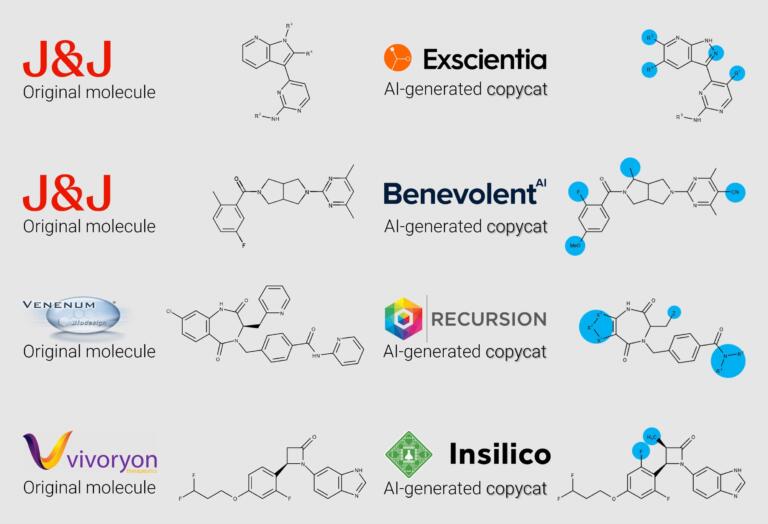
The End of the Line for Some…
We’re now seeing the start of a major shakeout in the AI-driven drug discovery space.
BenevolentAI’s stock has taken a pummeling over the past year or so, and they’ve had major layoffs. Ex-scientia’s days as an independent company recently ended when Recursion purchased them for $688 million – a fraction of their $2.9 billion market cap at IPO.
Did It Have to Be This Way?
The root of the problem is that Deep Learning, which underlies nearly all drug-discovery AI, was developed under the Big Data paradigm. This approach thrives when there is a copious amount of dense, high-quality data, but struggles when datasets are small and thinly populated.
What Data Do We Have?
Over the past 150 or so years, traditional pharmaceutical companies have produced and tested under ten million distinct families of chemicals in the lab. Whatever experimental data the traditional pharma companies made public was the dataset AI-driven companies inherited. But this dataset is problematic for several reasons.
It’s Actually Not That Much Data…
Experimental data for compounds from ten million chemical families sounds like a lot until you realize that there are, depending on whom you ask, a novemdecillion (or one followed by 60 zeroes) drug-like compounds that could be synthesized and tested under the rules of organic chemistry.
Does anyone really think ten million chemical families can form a representative sample for a set of a no-vemdecillion?
Remember, AI will only produce similar compounds to the examples on which it was trained.
…The Available Data Actually Isn’t That Good…
Unfortunately, there are also limitations on the quality of the experimental data we have in the life sci-ences. Half, if not more, of peer-reviewed life-science papers contain data that is flawed and cannot be reproduced. Bottom line: a big portion of the data we’re feeding drug-discovery AI is suspect.
…And We Have Almost No Data on “Failed” Experiments
There is very little data publicly available for compounds with negative experimental outcomes. Most organizations focus on publishing data for successful experiments. But data from “failed” experiments is as important for training valid AI models as data from “successful” ones. The “negative” examples are missing from drug-discovery AI’s dataset almost entirely.
But Wait, What About Generative AI?
Some companies are now hanging their hopes on using generative AI to help produce compounds that don’t have the same basic molecular structures as existing drugs.
But generative AI does not overcome one fundamental constraint: it cannot produce things that are rad-ically different from examples in the dataset on which it was trained.
Is There a Better Way Forward?
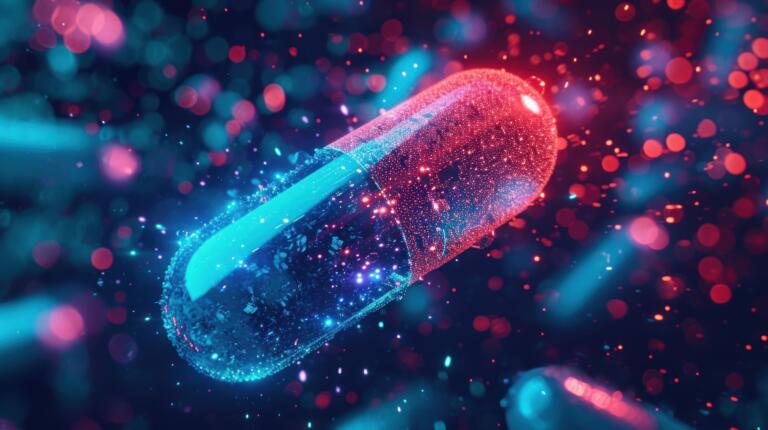
You might come away from this article with the impression that drug-discovery AI has hit a dead end. That’s the case only for companies that rely on an AI-only or AI-first approach. Using AI alone for funda-mental drug discovery is misusing AI, undercutting its true potential.
Accurate physics-based simulations can generate what current AI parlance calls “synthetic” data to dis-tinguish it from experimental data. AI trained on such synthetic data can surmount the limitations I’ve described.
Silicon Valley-based Verseon’s platform and drug pipeline seem to validate this approach. But how they overcame AI’s data problem is a subject for another article.
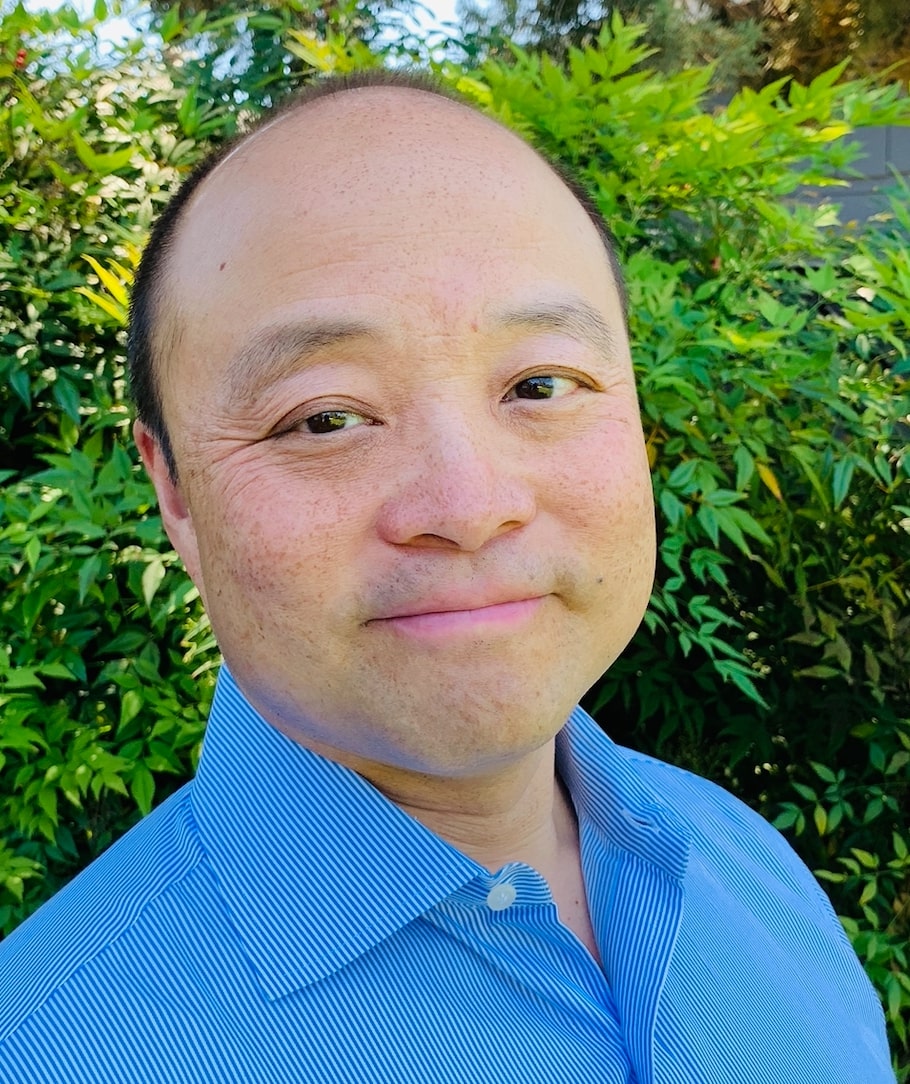
Brian Wang
Brian Wang is a Futurist Thought Leader and a popular Science blogger with 1 million readers per month. His blog Nextbigfuture.com is ranked #1 Science News Blog. It covers many disruptive technology and trends including Space, Robotics, Artificial Intelligence, Medicine, Anti-aging Biotechnology, and Nanotechnology.
Known for identifying cutting edge technologies, he is currently a Co-Founder of a startup and fundraiser for high potential early-stage companies. He is the Head of Research for Allocations for deep technology investments and an Angel Investor at Space Angels.
A frequent speaker at corporations, he has been a TEDx speaker, a Singularity University speaker and guest at numerous interviews for radio and podcasts. He is open to public speaking and advising engagements.