
The first article in this series interviewed companies that give patients a voice somehow in the development of AI models affecting health care. I talked to several other companies that try in various ways to improve the typical use of patient input developing AI.
“Don” Dongyang Zhang, MD/PhD, AI Lead for Elekta, describes the common technique called instruction tuning that refines existing AI models: “Human feedback, including from patients, is crucial in refining these responses: we can create, the old-fashioned way, what an ideal response should look like and then feed a set of such responses to the AI to allow it to learn by example and generate responses closer to the desired output.”
Avanade, now part of Microsoft, is an AI-based collaboration platform. In healthcare, it can help teams find solutions to patient problems; I recently remarked on their use for assessing social determinants of health.
Although Avanade has used patient responses as input to machine learning for some time, they have recently started interviewing patients to identify biases in health care systems.
edYOU also employs user surveys to improve its AI models, which feed its tutoring platform for wellness and other educational services. According to its CEO, Dr. Michael Everest, they gather feedback through surveys and interviews. He says, “The collaboration between patients, data scientists, and clinicians creates AI models that provide meaningful and impactful experiences for all involved.”
Everest describes how the data is incorporated as follows: “edYOU’s intelligent curation engine (ICE) provides a safe-walled garden for edYOU’s AI platform. Through ethical data curation and human oversight, ICE traces data origins, flags bias, and decentralizes provenance. ICE provides the standard for transparent and trustworthy AI. Coupled with feedback from interviews, testimonies, and surveys, edYOU’s Proprietary Ingestion Engine (PIE) intelligently processes the data and filters information to enable personalized education experiences.”
Altimetrik derives data from “an open-ended set of questions” to patients. Ramji Vasudevan, Senior Engineering Leader, warns about some of the biases introduced by asking patients for input: “Some patients are inherently optimistic or pessimistic, which creates a confidence bias. Using the same measure for their response, may sometimes yield inaccurate results from the AI model. The over/under information bias occurs again when some patients are more informed than others, leading to different interpretations of questions, especially abstract ones. This can also lead to inaccurate representation of data. The risk mitigation is to make the questions as unambiguous and direct as possible.”
Patient Data in Health Care AI
I believe there is an ethical imperative to get patient commentary and advice for AI, because when all is said and done, we’re using their data to develop the models, as well as trying to advise them on what to do.
Raman Sapra, President & Global Chief Growth Officer at the IT services company Mastek, says, “As AI models take an increasingly larger role across Healthcare, it’s imperative to integrate patient/public involvement in building these models. Having lived through a chronic condition or disease, patients’ inputs act as a catalyst that helps the AI models for diagnosing an ailment or during clinical trials to achieve a higher level of data accuracy. Including patient input also fosters building trust across patients and clinical It’s also important to demystify the AI models and democratize data.”
Some common uses of patient data in AI include the following:
- Interzoid offers data cleansing and usability, taking patient data from website forms, OCR processing of paper documents and questionnaires, and in other ways. Founder and CEO Bob Brauer says, “Healthcare data, including patient names, supplier names, provider names, product names, and many other types of data collected through surveys and other means are inherently inconsistent, making analysis and AI-model building tremendously difficult. It can often lead to inaccurate models, non-representative datasets, disjoint analysis, and errant decision-making. While numerical data quality issues are often very difficult to resolve, challenges resulting from inconsistent use and capture of natural language data can be overcome, usually with modest investment.”
- Apierion (formerly MAPay), which creates a “digital medical twin” combining a wide range of patient data, are starting to supplement the usual clinical and payer databases by collecting data from patients through wearables and SDoH characteristics of their environments. Michael “Dersh” Dershem, Founder and CEO, says they are also starting to reward patients to provide data. They are testing models for rewards, including partnerships with employers and awarding tokens that can be used to pay insurance premiums and make health care purchases.
- Phamily, a care management platform from Jaan Health, engages patients using conversational AI to prevent readmissions and provide other preventative care (Figure 1). According to Toufique Harun, Co-founder and Chief Product Officer, Phamily uses surveys and regular proactive check-ins to find out what services patients need and how the services can better enable patients to achieve their health goals. Patient responses are used as a feedback loop to improve patient engagement.
- Verseon uses AI along with quantum physics to design new drug compounds. They collect patient data during clinical trials, partly through self-reporting, which can “add significant additional insight into progression of disease conditions and effects of therapeutic intervention,” according to CEO Adityo Prakash. The data is “combined with additional objective biomarkers.”
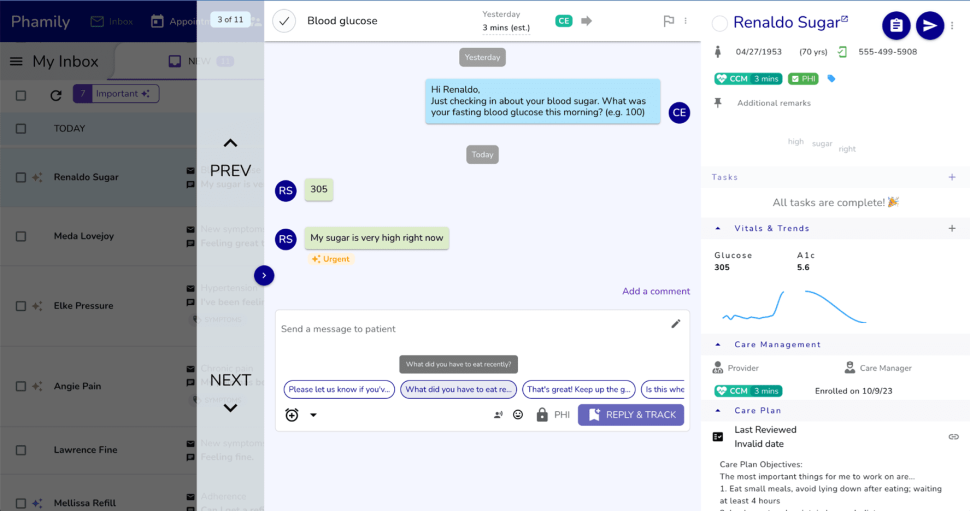
Should Patients Assess the AI That Assesses Them?
The use of patient data is nearly ubiquitous in health care AI. And even though we know that the models coming hot out of the AI oven tend to contain inaccuracies, very few AI firms provide a feedback path for patient input, even in this age of the slogan “Nothing about us without us.” As John Squeo, Senior Vice President & Market Head, Healthcare Providers at CitiusTech, says, “There is no direct feedback loop as to quality and trust.” Although CitiusTech does not use direct patient input to change AI models, its Health System clients frequently do have community members or patients on engagement committees, which could be used to gather patient sentiment concerning AI and new technologies.
Of course, taking patient input is labor-intensive. Data scientists using this input need to anticipate how to handle the influx of ideas and how they can apply the ideas to altering AI-generated models. I hope that his article inspires more companies to try.